Humanoid Robot Autonoumous Bimanual Manipulations
Humanoid Robot Autonoumous Bimanual Manipulations
Research Summary
The autonomous manipulation of highly redundant humanoid robots is a great challenge and potential topic. Compared with traditional single-arm robot learning, it needs to consider the coordination among multiple limbs (like bimanual arms) and even the whole body. This redundancy also provides a structural basis for the robot to learn manipulation skills in the human style, which is meaningful in domestic service tasks, especially in most kitchen scenarios. Since soft and deformable object manipulation is common in kitchen tasks, it becomes another intractable topic we are interested in, except for bimanual / whole-body coordination. Therefore, we propose combining multi-modal human demonstration and learning-based methods to achieve soft object manipulation with multi-limb coordination from learning the object representation and the relationship between robot manipulation and object state change.
Research 1 : Learning Dynamic Bimanual Coordinated Movements for Non-prehensile Manipulation from Human Demonstration
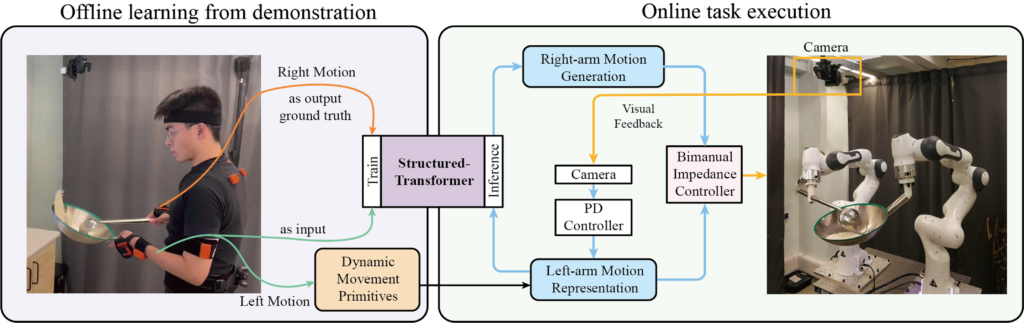
Stir-fry, the famous Chinese cooking art, requires a sequence of highly dynamic bimanual coordinated movements for manipulating non-prehensile contents. We propose a decoupled framework for learning this task from human demonstration. Dual arms of the robot are decoupled into different roles, then the bimanual task is transformed into a coordination problem, for which we propose a Graph and Transformer based model—Structured-Transformer, to capture the spatio-temporal relationship between dual-arm movements. By adding visual feedback of contents deformation, our framework adjusts the movements automatically to achieve the desired stir-fry effect.
J. Liu, Y. Chen, Z. Dong, S. Wang, S. Calinon, M. Li and F. Chen, “Robot Cooking with Stir-fry: Bimanual Non-prehensile Manipulation of Semi-fluid Objects,” IEEE Robotics and Automation Letters, vol. 7, no. 2, pp. 5159-5166, 2022.
Research 2 : Learning Goal-oriented Deformable Manipulation Policy with the Graph that Extracts the Object Dynamics and Manipulation Policy
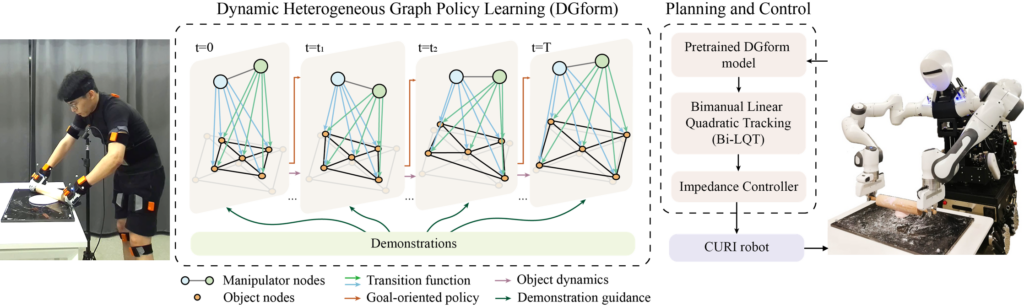
The state representation and manipulation policy learning are essential techniques for handling deformable objects. We propose a Dynamic heterogeneous Graph based model for learning Goal-oriented deformable manipulation policy, which uses the graph as a unified form of state representation and policy learning. From this graph, we extract the object dynamics and manipulation policy, and integrate the demonstration to guide policy learning. The method was deployed on the CURI robot for a bimanual dough rolling task from first-person perspective.
J. Liu, C. Li, R. Huang, Z. Li, S. Calinon and F. Chen, “DGform: Learning Goal-oriented Deformable Manipulation Policy via Dynamic Heterogeneous Graph,” To be submitted (2022).